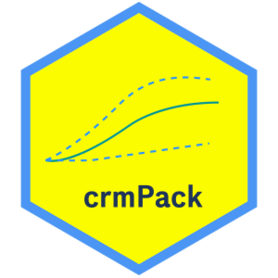
Summarize the dual-endpoint design simulations, relative to given true dose-toxicity and dose-biomarker curves
Source:R/Simulations-methods.R
summary-DualSimulations-method.Rd
Summarize the dual-endpoint design simulations, relative to given true dose-toxicity and dose-biomarker curves
Arguments
- object
the
DualSimulations
object we want to summarize- trueTox
a function which takes as input a dose (vector) and returns the true probability (vector) for toxicity.
- trueBiomarker
a function which takes as input a dose (vector) and returns the true biomarker level (vector).
- target
the target toxicity interval (default: 20-35%) used for the computations
- ...
Additional arguments can be supplied here for
trueTox
andtrueBiomarker
Value
an object of class DualSimulationsSummary
Examples
# Define the dose-grid.
emptydata <- DataDual(doseGrid = c(1, 3, 5, 10, 15, 20, 25, 40, 50, 80, 100))
# Initialize the CRM model.
my_model <- DualEndpointRW(
mean = c(0, 1),
cov = matrix(c(1, 0, 0, 1), nrow = 2),
sigma2betaW = 0.01,
sigma2W = c(a = 0.1, b = 0.1),
rho = c(a = 1, b = 1),
rw1 = TRUE
)
# Choose the rule for selecting the next dose.
my_next_best <- NextBestDualEndpoint(
target = c(0.9, 1),
overdose = c(0.35, 1),
max_overdose_prob = 0.25
)
# Choose the rule for stopping.
my_stopping1 <- StoppingTargetBiomarker(
target = c(0.9, 1),
prob = 0.5
)
# For illustration stop with 6 subjects.
my_stopping <- my_stopping1 | StoppingMinPatients(6) | StoppingMissingDose()
# Choose the rule for dose increments.
my_increments <- IncrementsRelative(
intervals = c(0, 20),
increments = c(1, 0.33)
)
# Initialize the design.
design <- DualDesign(
model = my_model,
data = emptydata,
nextBest = my_next_best,
stopping = my_stopping,
increments = my_increments,
cohort_size = CohortSizeConst(3),
startingDose = 3
)
# Define scenarios for the TRUE toxicity and efficacy profiles.
beta_mod <- function(dose, e0, eMax, delta1, delta2, scal) {
maxDens <- (delta1^delta1) * (delta2^delta2) / ((delta1 + delta2)^(delta1 + delta2))
dose <- dose / scal
e0 + eMax / maxDens * (dose^delta1) * (1 - dose)^delta2
}
true_biomarker <- function(dose) {
beta_mod(dose, e0 = 0.2, eMax = 0.6, delta1 = 5, delta2 = 5 * 0.5 / 0.5, scal = 100)
}
true_tox <- function(dose) {
pnorm((dose - 60) / 10)
}
# Draw the TRUE profiles.
par(mfrow = c(1, 2))
curve(true_tox(x), from = 0, to = 80)
curve(true_biomarker(x), from = 0, to = 80)
# Run the simulation on the desired design.
# For illustration purposes 1 trial is simulated with 5 burn-ins to generate 20 samples.
my_sims <- simulate(
object = design,
trueTox = true_tox,
trueBiomarker = true_biomarker,
sigma2W = 0.01,
rho = 0,
nsim = 1,
parallel = FALSE,
seed = 3,
startingDose = 6,
mcmcOptions = McmcOptions(
burnin = 5,
step = 1,
samples = 20
)
)
# Summarize the results of the simulations.
summary(
my_sims,
trueTox = true_tox,
trueBiomarker = true_biomarker
)
#> Summary of 1 simulations
#>
#> Target toxicity interval was 20, 35 %
#> Target dose interval corresponding to this was 51.6, 56.1
#> Intervals are corresponding to 10 and 90 % quantiles
#>
#> Number of patients overall : mean 3 (3, 3)
#> Number of patients treated above target tox interval : mean 0 (0, 0)
#> Proportions of DLTs in the trials : mean 0 % (0 %, 0 %)
#> Mean toxicity risks for the patients on active : mean 0 % (0 %, 0 %)
#> Doses selected as MTD : mean 0 (0, 0)
#> True toxicity at doses selected : mean 0 % (0 %, 0 %)
#> Proportion of trials selecting target MTD: 0 %
#> Dose most often selected as MTD: 0
#> Observed toxicity rate at dose most often selected: NaN %
#> Fitted toxicity rate at dose most often selected : mean NA % (NA %, NA %)
#> Stop reason triggered:
#> P(0.9 ≤ Biomarker ≤ 1) ≥ 0.5 (relative) : 0 %
#> ≥ 6 patients dosed : 0 %
#> Stopped because of missing dose : 100 %
#> Fitted biomarker level at dose most often selected : mean NA (NA, NA)